Coordinated Control and Task Allocation for Autonomous Mobile Robots in Warehouses using Multi-objective Multi- agent-Software
Research on Operational Efficiency focuses on Warehouse Robots Fleet Management MSMEs often rely on paper-based documentation for order tracking in addition to inventory management, purchase orders, invoices, quality assurance records, and regulatory compliance. This reliance on physical documents can lead to delays, lack of audit trails, errors, and data loss, ultimately affecting productivity and compliance with industry standards. Optical Character Recognition (OCR) which is an AI- based method, is being explored to digitize documents by simply scanning them using edge devices. This eliminates manual data entry, reducing errors and improving efficiency. OCR-powered AI can also categorize and integrate extracted data into digital systems, ensuring quick access to information while maintaining audit trails.
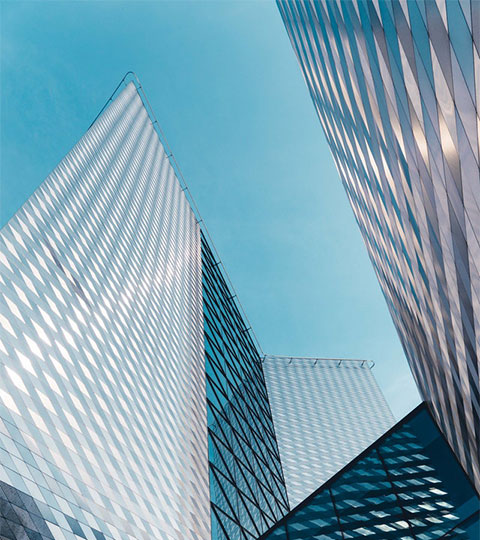
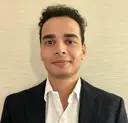
Key Features of the Project
Two-Stage Shelf Movement: Allows more efficient robot allocation compared to previous methods, where a single robot handled both picking and returning a shelf.
Dynamic Robot Utilization: A robot does not have to return the same shelf it delivered. It can pick up and return different shelves, optimizing robot usage.
Continuous Order-to-Task Conversion: Unlike traditional batch-based order fulfillment, this system continuously converts orders into tasks. Ensures that all shelf movements align with real-time order demands.
Dynamic Task Buffer: The system can modify existing tasks when a new order arrives. Allows real- time adaptation to changing order demands.
Warehouse Layout Independence: The system is designed to function efficiently across various warehouse layouts, making it highly scalable and adaptable.