Lightweight and Scalable AI for Manufacturing
Enhancing operational efficiency through piolt for inventory mangement at Acutech Pvt. Ltd. Manufacturing MSMEs face resource constraints, fragmented systems, and reliance on manual inspection, limiting quality control and efficiency. While AI-driven solutions offer improvements, high computational costs and technical expertise requirements hinder their adoption. To address this, we propose a lightweight, edge-deployable AI framework integrating real-time defect detection, robust anomaly identification (OOD detection on MVTec dataset), generative defect synthesis (for data augmentation in industrial settings), and deep learning model compression (adaptive quantization and pruning for low-power and edge deployment). This approach enables MSMEs to implement cost- effective, scalable AI solutions for defect detection, predictive analytics, and quality assurance while operating within resource limitations.
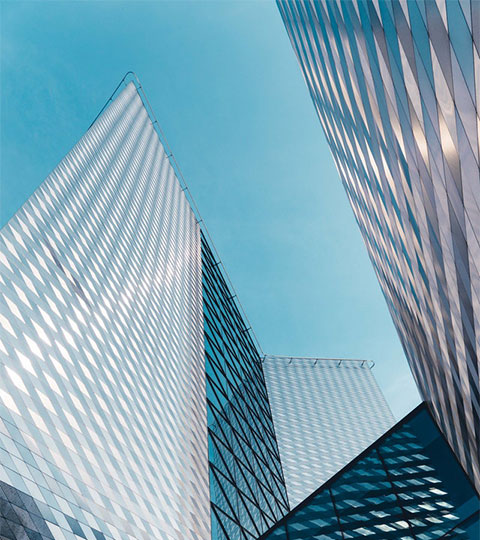
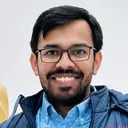
Key Features of the Project
This project focuses on deep learning model compression for manufacturing, enabling AI deployment on edge devices while maintaining accuracy. The key project components and innovations include:
Defect Detection using YOLOv8n on the NEU-DET Dataset: Classifies six types of steel surface defects for real-time quality control. Reduces human intervention, enhancing defect detection efficiency.
Out-of-Distribution (OOD) Detection on MVTec Dataset: Identifies anomalies beyond trained categories, improving robustness. Reduces false detections, ensuring reliable defect detection.
Generative Defect Synthesis for Industrial Anomaly Detection (Submitted @CVPRw2025): Uses generative models to create realistic defects for training. Enhances detection accuracy and reduces reliance on manual defect data.
Deep Learning Model Compression for Edge Deployment: (Accepted @NeurIPSw, WACVw, ICLRw, and CVPRw2025) n2Implements adaptive quantization and pruning for low-power devices. Maintains accuracy while significantly reducing computational costs. This project enables AI-driven defect detection and anomaly analysis in MSMEs. The lightweight framework ensures real-time quality control while being cost-effective and scalable for industrial applications.