RUL Informed Backordering Strategy Development for Induction Motor Windings
Improving Operational Efficiency, Asset Efficency, and Sustainbility through Development in Induction Motor Windings for the Foundry Sector In industries relying on induction motors, unexpected failures in motor windings can lead to costly downtime. Predictive maintenance strategies, especially Remaining Useful Life (RUL) estimation, play a crucial role in mitigating these failures. This project focuses on developing a backordering strategy for induction motor windings using RUL predictions, ensuring optimal inventory management for Micro, Small, and Medium Enterprises (MSMEs). By integrating condition monitoring techniques and machine learning models, the proposed approach minimizes unplanned stoppages and reduces operational costs. This research aims to enhance MSME sustainability by providing a data-driven solution for spare part procurement, improving efficiency, and extending motor lifespan.
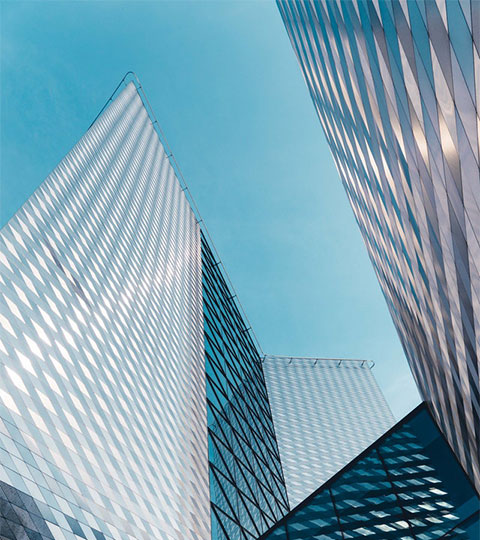
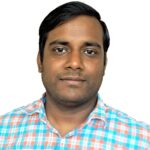
Key Features of the Project
This platform is purpose-built to meet MSME needs, focusing on simplicity, scalability, and practical usability. Key features include:
RUL-Based Backordering: Uses Remaining Useful Life (RUL) predictions to optimize spare part procurement, reducing inventory costs.
Condition Monitoring Integration: Leverages real-time data from sensors for accurate motor health assessment.
AI-Driven Predictive Analytics: Employs machine learning algorithms to forecast motor winding failures.
Automated Alerts & Recommendations: Notifies MSMEs about impending failures and suggests timely backordering actions.
Cost Optimization: Minimizes unplanned downtime and prevents overstocking or stockouts.
User-Friendly Dashboard: Provides an intuitive interface for monitoring motor health and managing spare parts.
Scalable & Customizable: Adapts to different industrial applications and business needs.